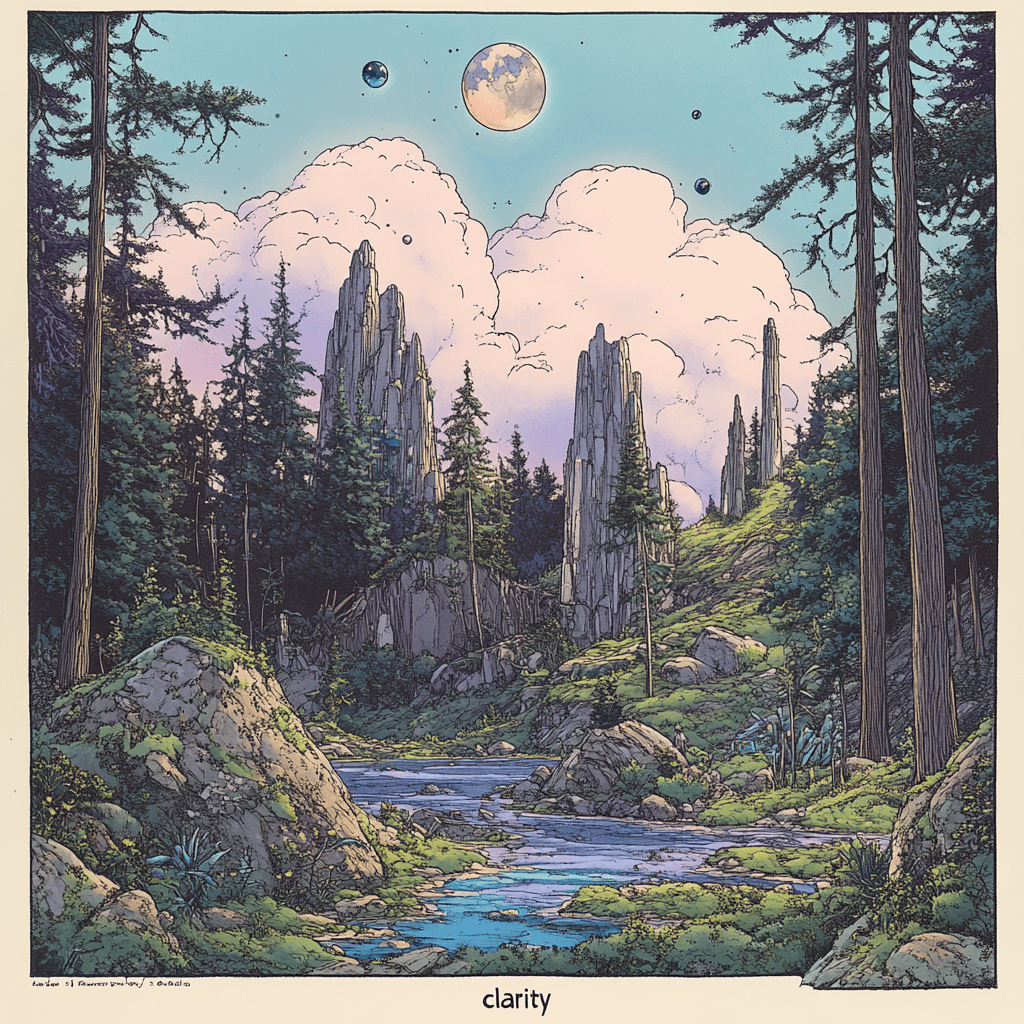
What Model Should I Use in Cline?
One of the most common questions developers ask when getting started with Cline is: "Which AI model should I use?" With Cline's approach to supporting virtually all models from the top providers (Anthropic, Google Gemini, OpenAI, and others), the choices can be overwhelming. The truth is, there's no single "best" model for all situations. What works brilliantly for one development task might be overkill – or underpowered – for another. Let's break down how to think about model selection through
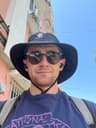
One of the most common questions developers ask when getting started with Cline is: "Which AI model should I use?" With Cline's approach to supporting virtually all models from the top providers (Anthropic, Google Gemini, OpenAI, and others), the choices can be overwhelming.
The truth is, there's no single "best" model for all situations. What works brilliantly for one development task might be overkill – or underpowered – for another. Let's break down how to think about model selection throughout the software development lifecycle, combining insights from benchmarks with practical cost considerations.
The Development Lifecycle Approach to Model Selection
Different phases of development demand different AI strengths. Here's how to approach model selection across a typical project:
Design & Architecture Phase
When you're starting a new project, it's tempting to always reach for the most popular or newest model. However, what you really need at this stage is a model with strong reasoning capabilities and a robust knowledge base to understand domain-specific business requirements.
What to look for: Strong chain-of-thought reasoning and general knowledge
Benchmark to consider: MMLU Pro, which scores models on their reasoning ability
Current strong performers:
- OpenAI o1 (GPT-4.5)
- Gemini 2.5 Pro
- DeepSeek R1 (671B)
Cost consideration: This is one phase where investing in a premium model often pays dividends. Clear architectural decisions made early can save weeks of rework later, making this a smart place to allocate AI budget.
Development Phase
During active coding, you need a model that excels at understanding code patterns, suggesting completions, and explaining implementations.
What to look for: Real-world coding performance, not just benchmark scores
Benchmark to consider: Community-driven leaderboards like Chatbot Arena, which shows how well models perform with actual users
Current strong performers:
- Gemini 2.5 Pro
- GPT-4o
- Grok 3
Practical insight: Interestingly, Claude 3.7 Sonnet remains highly sought-after by many developers despite not always scoring highest on standard benchmarks. This highlights the importance of trying multiple models rather than relying solely on benchmarks.
Cost consideration: For routine code completion and straightforward development tasks, you can often use mid-tier models without sacrificing much productivity. Save the premium models for complex implementation challenges.
Testing Phase
When writing tests, you need a model that excels at understanding edge cases and writing robust test code.
What to look for: Proficiency in coding tasks and challenges
Benchmark to consider: Big CodeBench
Current strong performers:
- Claude 3.7
- OpenAI o1
- GPT-4o Mini
Cost consideration: Since tests often run in isolation and follow common patterns, this is an area where mid-tier models can often perform adequately for simple test suites. Reserve premium models for complex testing scenarios or performance-critical code.
Deployment & Review Phase
By the time you reach deployment, you have substantial code to review and integrate. This phase benefits from models with large context windows that can understand your entire codebase.
What to look for: Large context windows and multimodality (for reviewing screenshots, diagrams, etc.)
Benchmark to consider: MMMU for multimodality
Current strong performers:
- Gemini 2.5 Pro
- GPT-4o Mini
- GPT-4.1
- OpenAI o1
Cost consideration: The ability to process your entire codebase in one go can significantly speed up review cycles. This is another area where premium models with larger context windows often justify their cost through time savings.
Beyond Benchmarks: Practical Tips for Finding Your Ideal Model
While benchmarks provide useful guidance, here are some additional tips that can help:
- Start with a mid-tier model and upgrade when needed. Many development tasks don't require the most expensive options. Begin with something like Claude 3 Haiku or GPT-3.5 and switch to premium models only when you hit limitations.
- Create model presets for different tasks. In Cline, you can set up different model configurations for different types of work. Consider creating presets for brainstorming (premium model), routine coding (mid-tier), and documentation (budget-friendly).
- Watch your token usage. Cline's token counter helps you identify where you're spending most of your AI budget. Use this data to optimize which models you use for frequent tasks.
- Remember that benchmarks are relative. They show how models perform against each other but may not reflect your specific use case. Always contextualize benchmark results against what you're trying to accomplish.
- Experiment during non-critical phases. Use downtime or personal projects to test different models and build intuition for which ones excel at various tasks.
- Consider different models for Plan and Act modes. Cline's Plan/Act workflow lets you use different models for different modes. Many developers choose stronger reasoning models like Gemini 2.5 Pro for planning tasks, then switch to faster, more economical models like Gemini 2.5 Flash Preview for implementation.
The ideal approach combines a mix of benchmarks and experimentation. With Cline's flexibility to switch between models, you can find the perfect balance of performance and cost efficiency for each stage of your development workflow.
Join our Discord or Reddit communities to share your experiences with different models and learn from other developers about which combinations work best for various project types.
This blog was written by Nick Baumann, Product Marketing at Cline. Follow us @cline for more insights into the future of development.